Machine Learning Model Serving Patterns and Best Practices
Machine Learning Model Serving Patterns and Best Practices
Regular price
Checking stock...
Regular price
Checking stock...
Summary
Mastering the art of model serving is a crucial yet daunting task in the realm of machine learning. This comprehensive guide equips you with knowledge of various model serving techniques to seamlessly bring your machine learning models to fruition, while also highlighting the best practices in model deployment.
The feel-good place to buy books
- Free delivery in the UK
- Supporting authors with AuthorSHARE
- 100% recyclable packaging
- Proud to be a B Corp – A Business for good
- Buy-back with Ziffit
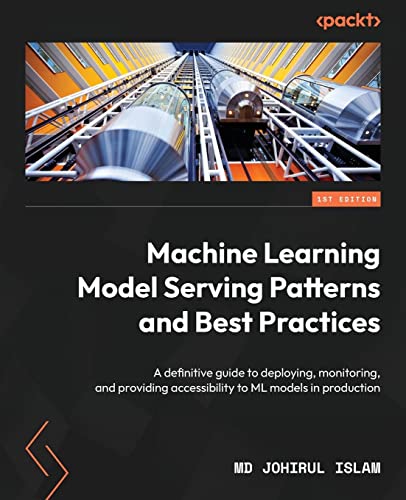
Machine Learning Model Serving Patterns and Best Practices by Md Johirul Islam
Become a successful machine learning professional by effortlessly deploying machine learning models to production and implementing cloud-based machine learning models for widespread organizational use Key Features Learn best practices about bringing your models to production Explore the tools available for serving ML models and the differences between them Understand state-of-the-art monitoring approaches for model serving implementations Book DescriptionServing patterns enable data science and ML teams to bring their models to production. Most ML models are not deployed for consumers, so ML engineers need to know the critical steps for how to serve an ML model. This book will cover the whole process, from the basic concepts like stateful and stateless serving to the advantages and challenges of each. Batch, real-time, and continuous model serving techniques will also be covered in detail. Later chapters will give detailed examples of keyed prediction techniques and ensemble patterns. Valuable associated technologies like TensorFlow severing, BentoML, and RayServe will also be discussed, making sure that you have a good understanding of the most important methods and techniques in model serving. Later, you'll cover topics such as monitoring and performance optimization, as well as strategies for managing model drift and handling updates and versioning. The book will provide practical guidance and best practices for ensuring that your model serving pipeline is robust, scalable, and reliable. Additionally, this book will explore the use of cloud-based platforms and services for model serving using AWS SageMaker with the help of detailed examples. By the end of this book, you'll be able to save and serve your model using state-of-the-art techniques. What you will learn Explore specific patterns in model serving that are crucial for every data science professional Understand how to serve machine learning models using different techniques Discover the various approaches to stateless serving Implement advanced techniques for batch and streaming model serving Get to grips with the fundamental concepts in continued model evaluation Serve machine learning models using a fully managed AWS Sagemaker cloud solution Who this book is forThis book is for machine learning engineers and data scientists who want to bring their models into production. Those who are familiar with machine learning and have experience of using machine learning techniques but are looking for options and strategies to bring their models to production will find great value in this book. Working knowledge of Python programming is a must to get started.
Md Johirul Islam is a Data Scientist and Machine Learning Researcher at AWS. He has a PhD in Computer Science and is also an adjunct professor at Purdue University. His expertise are focused on designing explainable, maintainable and robust data science pipeline applying the software design principles and helping organizations deploy Machine Learning models into production at Scale.
SKU | Unavailable |
ISBN 13 | 9781803249902 |
ISBN 10 | 1803249900 |
Title | Machine Learning Model Serving Patterns and Best Practices |
Author | Md Johirul Islam |
Condition | Unavailable |
Binding Type | Paperback |
Publisher | Packt Publishing Limited |
Year published | 2022-02-25 |
Number of pages | 336 |
Cover note | Book picture is for illustrative purposes only, actual binding, cover or edition may vary. |
Note | Unavailable |